Research Project Scope
Sri Lanka's economy heavily relies on agriculture, accounting for 45.46% of the nation's total land area in 2020. The World Bank's statistics on agricultural land in March 2023 provide current, historical, projections, and predictions. 30% of Sri Lankans work in the agricultural sector, despite the tropical nation's potential for crop growth. However, productivity and profitability issues hinder the sector's growth. To assist bell pepper growers in increasing crop yields and lowering losses, the PepperSmart programme employs a smart polytunnel technology. It employs machine learning to enable early identification and treatment of powdery mildew diseases via a smartphone application. Powdery mildew susceptibility rises with age in bell pepper plants, with older plants and mature lower leaves exhibiting the earliest signs. Live host plant tissue is necessary for the fungus' development and survival. Fluffy white dots on the underbelly that might eventually become brown are early indications. By putting the leaf up to the light and keeping an eye on the mildew colonies, you may quickly identify early powdery mildew infections. This research led to the creation of a smart polytunnel system that can detect and assess plant powdery mildew disease in its early stages. With the use of this technology, crop yields and quality may be improved, increasing sustainability and profitability. The method helps ensure that bell pepper producers and the agricultural industry have a more secure and prosperous future by enabling proactive and well-informed crop management decisions.
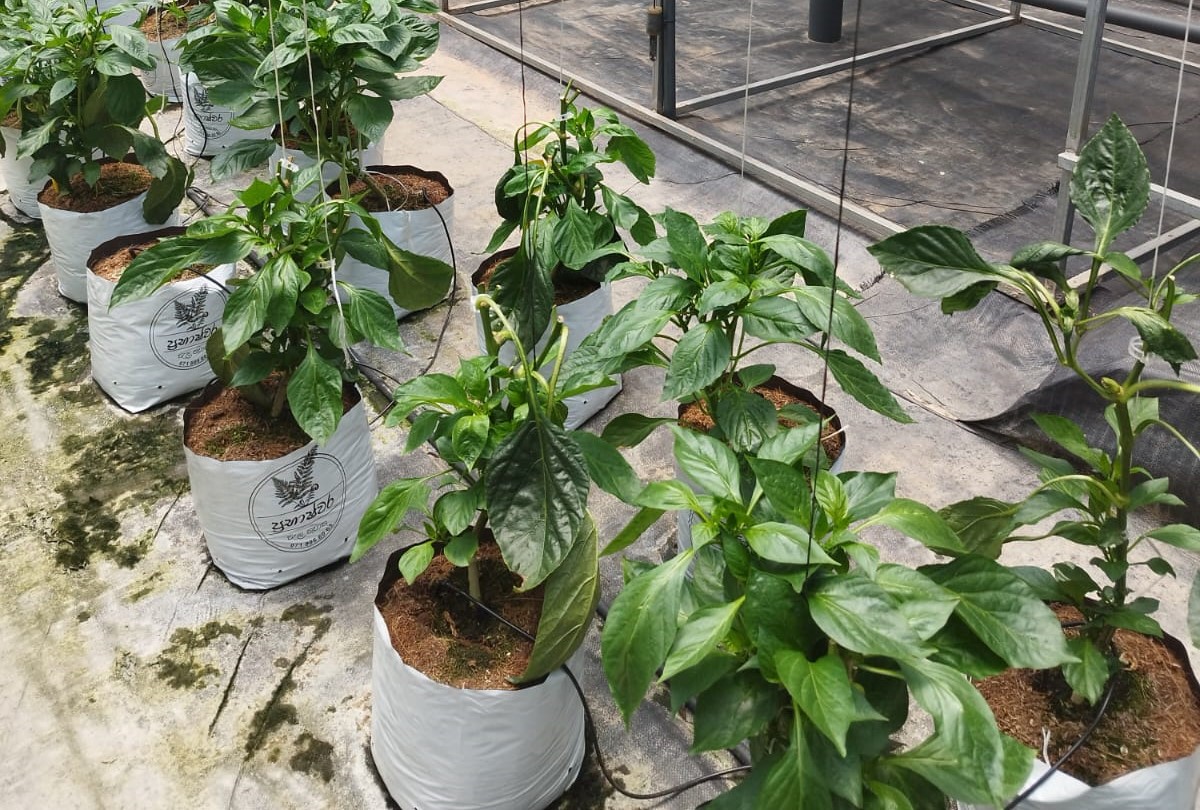
Literature Survey
Computer vision technology aids in identifying plant infection by capturing images and detecting pests and diseases, replacing outdated methods in agriculture(4). Plant infection, which are caused by elements like temperature, bacteria, and surrounding crops and manifest early through physical changes in plants, interrupt important processes and are harmful to their health(5)(6).Plant diseases have an effect on plant development and food supply(6). Research studies use a variety of techniques to discover plant diseases, influencing development and identifying variables affecting plant growth. In academic studies, plant diseases are identified and categorised using feature extraction, image processing, and SVM classifiers. These articles also address the issue of unbalanced data and investigate transfer learning methodologies for edge computing solutions(6)(7). A computer vision system that detects plant illnesses has a 93% accuracy rate and an average F1 score of 0.93. This strategy is computationally efficient and more precise than alternative approaches because it makes use of statistical image processing and machine learning models. For experts in vegetable pathology and pattern recognition, this article offers a thorough introduction(8)(9). Deep learning was used by researchers in 2021 to better diagnose plant diseases using a variety of ML techniques.(10). For the analysis and detection of plant leaf diseases, K.M. Mamatha, Seema Sing, and S.A. Hariprasad presented a convolutional neural network. The network examines pictures using a variety of strategies, concentrating on the leaves that are most susceptible to strain. Leaf samples are used as the dataset. For reliable results, pre-processed characteristics are used(11). A CNN method for diagnosing plant diseases using image processing was created by Sinha and Shekhawat in 2020. To detect NLB lesions in cropped photos, they developed a heat map and trained several CNNs(12). Four deep learning models that were trained on plant leaves fared better than earlier techniques in terms of accuracy and loss while identifying plant diseases(13). Sri Lanka's agriculture sector is essential for generating employment and ensuring food security. Secure bioconditions are necessary for the growth of healthy plants and the avoidance of disease in crops cultivated in polytunnels(14). Farmers depend on the leaf area index, but hand counting is time-consuming and challenging, hence automated technological alternatives are being investigated(15). Accurate ecophysiological evaluations and gas exchange in mid-latitude forests and shrublands need leaf tissue area (LAI). Polytunnels offer crop protection, however it might be difficult to maintain a secure bio environment. Automation for assessing plant health and boosting output is made possible by robotics, sensors, and AI technology. These instruments support problem-solving, damage minimization, and crop health surveillance. Robots using cameras can classify weeds, use less pesticides, and keep an eye on the health of crops to stop the spread of disease. The digital agricultural revolution has transformed farming practises, boosting output and having positive effects on the environment. Modern tools like sensors, GPS monitoring, and drones allow farmers to get real-time data on weather patterns, soil moisture, and crop growth rates. This Information enhances insect control, fertilisation, and irrigation, increasing crop yields and profitability. Efficiency and innovation are fostered by digitalization, which is fueled by trends like IoT and precision agriculture(16). In recent years, a number of venues for knowledge exchange have appeared. Information sharing platforms have advanced significantly as a new type of sharing economy, giving users additional avenues for Information acquisition, knowledge sharing, and personal knowledge storage. However, there are still issues with knowledge sharing platforms, such as inconsistent product quality (Content quality) and bad user experience(17). By encouraging Information exchange and agricultural innovation among farmers, professionals, academics, lawmakers, and consumers, social media helps to break down regional barriers and strengthen global connectivity. The most recent research has made progress in using computer vision to detect plant diseases. On the other hand, there is an enormous deficit in knowledge about the early diagnosis of powdery mildew in bell pepper crops by detecting the stressed level. While several research have looked into disease identification using deep learning and image processing, little focus has been placed on the unique difficulties posed by powdery mildew in bell pepper plants. By creating and evaluating the "PepperSmart" smart polytunnel system, which is particularly designed for the prompt detection and treatment of powdery mildew in bell pepper farming by recording the stressed level of the leaf, the current study aims to close this discrepancy. This targeted effort has the potential to promote sustainable farming methods inside Sri Lanka's crucial agriculture industry.
Research Gap
A significant body of research has explored various aspects of powdery mildew in bell pepper plants, including epidemiology, symptomatology, and treatment. However, several research gaps still exist, particularly regarding the use of advanced image analysis methods, such as Convolutional Neural Networks (CNN), Mask R-CNN, and YOLOv8, to assess the degree of illness and the application of agrochemicals in bell pepper plants. While these deep learning techniques have shown promise in identifying and measuring plant diseases in other crops, there's a lack of comprehensive evaluation of their efficacy in bell pepper plants. Additionally, AI models and mobile apps have been developed to automate powdery mildew detection in bell pepper plants. This research highlights the potential of using CNN, Mask R-CNN, and YOLOv8 for assessing the severity of powdery mildew in bell pepper plants based on lesion patterns in photos. The proposed solution includes the development of a mobile application to enhance accessibility. Furthermore, it mentions "Smart Pepper's" API access, which allows other parties to interact with the services. The research emphasizes the importance of accurate methods in training the algorithm and suggests a pro version of the solution for achieving the highest accuracy. The lack of early detection of powdery mildew in bell pepper plants by first assessing plant stress is identified as a research gap. The paper also compares this work with other research papers in the field, highlighting the shift from traditional image processing and machine learning techniques to deep learning methods for plant disease and pest detection. Several studies using deep learning techniques, including CNN, InceptionV3, InceptionResnetV2, MobileNetV2, EfficientNetB0, and SVM, have been cited for identifying various plant diseases and pests in different crops, demonstrating the versatility of deep learning in this context. Two additional studies outside the powdery mildew context focus on robotics, machine learning, and computer vision for plant monitoring in polytunnels. The first paper discusses the use of robotics and machine learning for disease identification and treatment in polytunnels using thermal imaging, while the second paper emphasizes estimating the leaf area index (LAI) of maize plants in polytunnels with machine learning and computer vision techniques.
Research Problem
Early detection of plant infection is essential for ensuring healthy plant development and, eventually,
for boosting agricultural output and food production. The risk of agricultural failure and loss can be
reduced by early discovery of plant illnesses, which can stop the disease from spreading to other plants
in the same area or adjacent fields. Furthermore, early discovery can reduce the need for pricey and
possibly dangerous chemical treatments, like pesticides and fungicides, which can have detrimental effects
on the ecosystem and human health.
Farmers and researchers can better understand the root causes of plant diseases and create efficient
management strategies to stop future outbreaks by spotting them in the early phases. This could involve
cultivating products that are immune to disease, enhancing soil management techniques, and using
pesticides and other remedies more effectively and strategically.
Identifying the progress level of "powdery mildew" disease in bell pepper plant and showing the plant area
in the map where agrochemical should be given for that disease according to the progress level of the
disease with poly-tunnel map
We have shared this link with bell pepper farmers who grow bell pepper inside polytunnels.
Here is the link :
Research Objective
The main objective of this research is to develop a mobile app with a function that will aid bell pepper growers in the early detection of "powdery mildew" infection. Powdery mildew is a fungal disease that affects many crops, including bell peppers, and can cause significant damage to the crop if left untreated. Therefore, this application will become a great help for bell pepper farmers to identify the disease during the early stage and this application will suggest suitable medications also.
The purpose of the mobile application is to assist farmers in detecting powdery mildew early on so they can respond quickly and stop the infection from infecting additional plants. The application may make use of image processing and object detection technologies to find the powdery mildew's distinctive signs.
A mobile application might help farmers save time and money by decreasing the need for costly and toxic pesticides by spotting powdery mildew early. The software may also assist farmers in increasing production and growing healthier crops, which would result in higher profitability and a more sustainable agricultural sector.
Methodology
The “PepperSmart” mobile application system is developed using 4 major components which are,
-
PepperSmart is a smart polytunnel application designed
for bell pepper farmers, offering disease detection, severity
detection, plant leaf area index detection, and post shearing
and commenting. It detects powdery mildew disease and rec-
ommends suitable medications for infected plants, enhancing
yield and cost. This application is crucial for preventing
powdery mildew and improving the overall quality of bell
pepper plants.
An infrared camera can identify the presence of powdery mildew by detecting stressed temperature. For early detection, high-resolution photos are essential. The software analyses each plant individually to measure stress levels and leaf area indices. The tool establishes a platform for Information exchange and use Auto ML to identify bell pepper plants that are disease-infected. The objective is to build a robot that can measure temperature and take pictures in order to diagnose and cure the illness.
This research is proposed as a smart solution to the main four problems identified in the agricultural sector in Sri Lanka. Once the application is developed, it will be introduced to bell pepper farmers, industry experts, and researchers to use it in their affairs. This application will be a great help to bell pepper farmers to maximize the crop yield and the income they get.

This study offers the bell pepper producers a rare chance to address their issues while maximizing their yields and revenue. The technology can be improved upon and modified for different products, dealing with more business issues.
A. Stressed Level Detection & Powdery Mildew Disease Detection of Bell Pepper Leaves
This section focuses on a complete method that combines temperature data analysis and picture classification techniques to evaluate the health state of bell pepper plants. Two primary duties are noted,
(1) Using temperature data to determine the degree of leaf stress.
(2) Using image classification to distinguish between healthy and sick bell pepper leaves.
Readings of the leaf temperature are gathered, and stress levels are calculated using a predefined threshold. To classify leaves into healthy and unhealthy categories, machine learning or deep learning models are applied. Combining these method- ologies enables a thorough evaluation of plant health and the creation of powdery mildew infection management strategies.
deep learning models were trained using temperature data and photos of bell pepper leaves. An extensive collection of leaf photos was captured with a high-resolution camera. The temperature of the bell pepper plants and their surroundings were continually measured using a thermal model. By examining temperature data, the study focuses on deter- mining the stress levels in bell pepper leaves. To measure stress levels, the researchers utilised a preset threshold or algorithm. The photographs were shot in a polytunnel, which controlled the development of bell peppers at various phases. The photos were carefully chosen to capture the leaves precisely, and using image classification, stressed and healthy leaves were distinguished.
Photographs of bell pepper leaves are manually annotated by plant health specialists to look for symptoms of stress and the disease powdery mildew. Machine learning algorithms or deep learning models are used to categorise leaves into groups that are healthy or powdery mildew-infected, image classification algorithms discern between healthy and diseased leaves. The first test focused on disease diagnosis and the second on identifying physiological stress. Combining these approaches offers a thorough assessment of bell pepper plant health, assisting in efficient management strategies.
By examining temperature data, the study focuses on determin- ing the stress levels in bell pepper leaves. To measure stress levels, the researchers utilised a preset threshold or algorithm. Various stages of bell pepper growth were regulated in the polytunnel. The photos were carefully chosen to capture the leaves and using image classification, stressed and healthy leaves were distinguished. The study used a convolutional neural network (CNN) archi- tecture to analyse the photos and categorise powdery mildew infection using TensorFlow, a machine learning framework. This technique made it easier to recognise stressed leaves and determine the degree of powdery mildew occurrence, which helped with a thorough evaluation of plant health.
B. Severity Calculation of infected bell pepper Leaves
The recommended method outlines an organised approach for assessing the severity of powdery mildew on bell pepper plants. The association between infection levels and yield loss is explored after a detailed analysis of the background Information, with an emphasis on the need of early detection. A detailed analysis of sickness symptoms and their repercus- sions emphasises the need of employing effective treatment methods.
The method then moves on to state-of-the-art technolog- ical choices, including TensorFlow and OpenCV-based AI- accelerated technologies that enable accurate severity analysis and pesticide optimisation. This serves as the foundation for the severity calculation approach, which is developed utilising methods like colour segmentation.
Automated chemical administration and disease progression assessment are crucial for accurate disease management. A smartphone app called ”Smart Poly Tunnel Helpful Guide” uses image recognition and model integration for real-time evaluation, interactive maps, and educational content. The strategy prioritizes user feedback and offers economic and ecological benefits, highlighting the potential for affordable chemical uses beyond agriculture.
As a result, this thorough methodology provides a compre- hensive approach that integrates user involvement, technolog- ical innovation, and economic factors to provide a dependable answer for estimating the severity of powdery mildew in bell pepper plants while laying the foundation for upcoming improvements and advancements.
C. Creating a Knowledge Sharing Platform using Posts
Develop a user interface that allows users to compose and format text posts. Use rich text editors to enable text formatting and media embedding. Implement the functionality to upload images along with posts, enabling users to share visual content
Allow users to add relevant tags to their posts. Use dropdowns or text inputs for tag entry. Create a system that categorizes posts based on user-assigned tags, making it easier for users to find and discover content.
Design an admin panel that allows moder- ators to review and approve posts before they become publicly visible. Implement automated content moderation algorithms to detect and flag inappropriate content, helping maintain a positive community environment.
D. Calculating the Leaf Area Index
Implement image processing techniques within the simulation to mimic real-world leaf detection. Apply color-based segmentation methods to identify leaves from the background.
Calculate the leaf area by sum- ming the areas of all detected leaf segments, considering the scale and resolution of the simulation.
Overlay the detected leaf segments on the simulation, showing users where the System has identified leaves. Provide a dynamic display of the calculated Leaf Area Index (LAI) as the simulation progresses, allowing users to see changes in real time.
This study offers the bell pepper producers a rare chance to address their issues while maximizing their yields and revenue
Technologies Used
Using Python models were trained and to implemented the application. The Jupyter Notebook is used to train models. React Native is used to implement the frontend (mobile application). The “Visual Studio Code” IDE is used to the development of the react native application.
Field Visits
We visited Modern Agri - Kadawatha





We visited Bandarawela to Collect Our Dataset
References
(4) X.W. Jun Liu, "Plant diseases and pests detection based on deep learning: a review," Plant Methods, 2021.
(5) p.K.A.D.P.B.a.Q.W. Jean B. Ristaino, "The persistent threat of emerging plant disease pandemics to global food security," PNAS, 2021.
(6) D.F.M.R.H.N. Soo Jun Wei, "Comparative study on the performance of deep learning implementation in the edge computing: Case study on the plant leaf disease identification," Journal of Agriculture and Food Research, 2022.
(7) M.S. ,.P.K.S. Anjna, "Hybrid System for Detection and Classification of Plant Disease," Hybrid System for Detection and Classification of Plant Disease, p. 1056–1065, 2020.
(8) A.K.T.K. ,. S.K.A.M.W. Pranesh Kulkarni, "Plant Disease Detection Using Image Processing and Machine Learning".
(9) A.B. Jayme Garcia, "Digital image processing techniques for detecting, quantifying and classifying plant," SpringurePlus, 2013.
(10) K.K.W.A.A.M.Q. Rehan Ullah Khan, "Image-Based Detection of Plant Diseases: From Classical Machine Learning to Deep Learning Journey," WILEY Hindawi, vol. 2021, p. 13, 2021.
(11) S.S.S.A.H.K.R. Mamatha, "Detection and Analysis of Plant Leaf Diseases Using Convolutional Neural Network," Journal of Computational and Theoretical Nanoscience, vol. 17, pp. 1-5, 2020.
(12) R.S.S. Aditya Sinha, "Review of image processing approaches for detecting plant diseases," IET Image Processing, vol. 14, no. 8, pp. 1427-1439, 13th May 2020.
(13) A.K.M.M.J.Z.L.E.J. Sk Mahmudul Hassan, "Identification of Plant-Leaf Diseases Using CNN and Transfer-Learning Approach," MDPI, vol. 10, no. 12, 9 June 2021.
(14) L.S.Y.L.J. , W.X.Zhang, "Leaf Area Index Estimation Using Convolutional Neural Network from UAV Images in Maize Field," in Journal of Sensors, 2021.
(15) N.H.E.-A.J.A. , . R.-P.J.R. Velez-Rivera, "Autonomous robot for plant monitoring and disease detection in protected environments," in Computers and Electronics in Agriculture, 2020.
(16) J. McFadden, F. Casalini, T. Griffin, and J. Antón, “The digitalisation of agriculture : A literature review and emerging policy issues | OECD Food, Agriculture and Fisheries Papers | OECD iLibrary,” no. April, pp. 1–57, 2022, [Online]. Available: https://www.oecd-ilibrary.org/content/paper/285cc27d-en https://www-oecd-ilibrary-org.libezproxy.open.ac.uk/agriculture-and-food/the-digitalisation-of-agriculture-285cc27d-en
(17) Shichao Pang, Peng Bao, Wenyuan Hao, and Jaewoong Kim, “Knowledge Sharing Platforms: An Empirical Study of the Factors Affecting Continued Use Intention,” in MDPI, vol. 12, no. 6, 17th March 2020.